Note: Today’s materials were developed for and borrowing from:
Create a repository called my-project
. This is just like we did this morning. It’s good to create new repos for new projects.
Please be sure to tick the box to Initialize this repository with a README. Otherwise defaults are fine.
Create a branch called gh-pages
.
This morning we were able to view our rendered .html pages online with rawgit. But a gh-pages
branch lets you have a clean url, which will be http://USER.github.io/REPO
(see more info at pages.github.com and User, Organization, and Project Pages - Github Help).
Set the default branch to gh-pages
, NOT the default master
.
Delete the branch master
, which will not be used.
Do this with RStudio if it’s working for you, or with the GitHub Desktop App.
Let’s learn markdown by editing the README.md
on github.com for convenience. A README.md file can be added to every folder in a repository, and they are automatically displayed when the repository is opened on github.com
The README.md
is in markdown, simple syntax for conversion to HTML. .md
is a kind of text file, so you only need a text editor to read it. If you were editing this on your computer, you could do this right in RStudio, which has a built-in text editor. (You could also do it in another text editor program, but RStudio is convenient). Copy-paste the following into your README.md
:
# my-project
Playing with [Software Carpentry at Oxford](http://jule32.github.io/2016-07-12-Oxford).
## Introduction
This repository demonstrates **software** and _formats_:
1. **Git**
1. **Github**
1. _Markdown_
1. _Rmarkdown_
## Conclusion
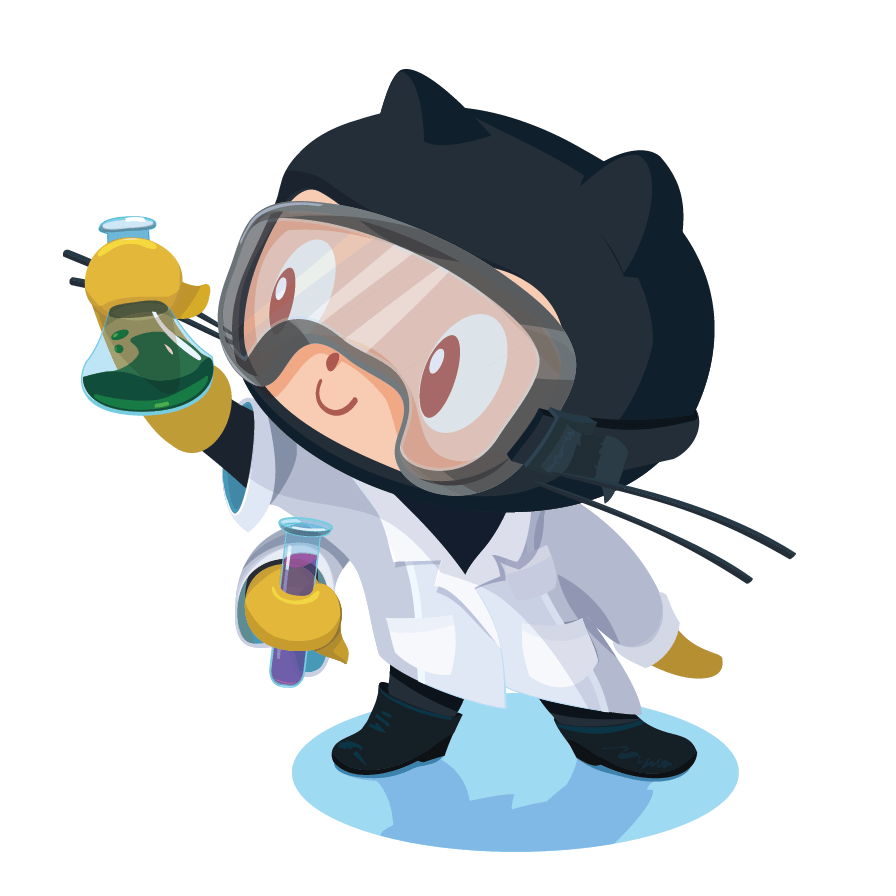
Now click on the Preview button to see the markdown rendered as HTML.
Notice the syntax for:
1.
, 1.
#
, ##
[](http://...)

_word_
**word**
There are some good cheatsheets to get you started, and here is one built into RStudio:
See Mastering Markdown · GitHub Guides and add some more personalized content to the README of your own, like a bulleted list or blockquote. For on the fly rendering, the atom text editor is good.
Have a look through the repo on github.com to see the updates you’ve made, and notice that it renders below the files in the repo. This is a unique trait of README.md
Back in RStudio, let’s create a new Rmarkdown file, which allows us to weave markdown text with chunks of R code to be evaluated and output content like tables and plots.
File -> New File -> Rmarkdown… -> Document of output format HTML, OK.
You can give it a Title of “My Project”. After you click OK, most importantly File -> Save as index
(which will get named with the filename extension index.Rmd
).
Some initial text is already provided for you. Let’s go ahead and “Knit HTML”.
Notice how the markdown is rendered similar to as before + R code chunks are surrounded by 3 backticks and {r LABEL}
. These are evaluated and return the output text in the case of summary(cars)
and the output plot in the case of plot(pressure)
.
Notice how the code plot(pressure)
is not shown in the HTML output because of the R code chunk option echo=FALSE
.
Before we continue exploring Rmarkdown, let’s sync this the .rmd and .html to github.com. Enter a message like “added index” and click on “Commit and Sync gh-pages”. This will update https://github.com/USER/my-project, and now you can also see your project website with a default index.html
viewable at http://USER.github.io/my-project
Were you hoping for an RStudio Cheatsheet? Here it is:
Let’s start a new .Rmd file and we’ll do this within an R chunk.
Great resources:
ggplot2
Let’s plot the population of countries in a single year of the gapminder dataset.
## load libraries
library(dplyr) # you need to have all libraries loaded within the Rmd file
library(ggplot2) #install.packages('ggplot2')
library(gapminder)
# get range of available data
summary(gapminder)
# setup dataframe
g = gapminder %>%
filter(year==2007) %>% # most recent year
mutate(pop_m = pop/1e6) # population, millions
# plot scatterplot of most recent year
s = ggplot(g, aes(x=gdpPercap, y=lifeExp)) +
geom_point()
s
# add aesthetic of size by population
s = s +
aes(size=pop_m)
s
# add aesthetic of color by continent
s = s +
aes(color=continent)
s
# add title, update axes labels
s = s +
ggtitle('Health & Wealth of Nations for 2007') +
xlab('GDP per capita ($/year)') +
ylab('Life expectancy (years)')
s
# label legend
s = s +
scale_colour_discrete(name='Continent') +
scale_size_continuous(name='Population (M)')
s
Your Turn: Make a similar plot but for gdpPercap
. Be sure to update the plot’s aesthetic, axis label and title accordingly.
# boxplot by continent
b = ggplot(g, aes(x=continent, y=lifeExp)) +
geom_boxplot()
b
# match color to continents, like scatterplot
b = b +
aes(fill=continent)
b
# drop legend, add title, update axes labels
b = b +
theme(legend.position='none') +
ggtitle('Life Expectancy by Continent for 2007') +
xlab('Continent') +
ylab('Life expectancy (years)')
b
plotly
library(plotly) # install.packages('plotly')
# scatterplot (Note: key=country shows up on rollover)
s = ggplot(g, aes(x=gdpPercap, y=lifeExp, key=country)) +
geom_point()
ggplotly(s) # must comment out or have eval=FALSE to knit
# boxplot
ggplotly(b) # must comment out or have eval=FALSE to knit
Your Turn: Expand the interactive scatterplot to include all the other bells and whistles of the previous plot in one continuous set of code (no in between setting of s).
The googleVis
package ports most of the Google charts functionality.
For every R chunk must set option results='asis'
, and once before any googleVis plots, set op <- options(gvis.plot.tag='chart')
.
suppressPackageStartupMessages({
library(googleVis) # install.packages('googleVis')
})
## Creating a generic function for 'toJSON' from package 'jsonlite' in package 'googleVis'
op <- options(gvis.plot.tag='chart')
m = gvisMotionChart(
gapminder %>%
mutate(
pop_m = pop / 1e6,
log_gdpPercap = log(gdpPercap)),
idvar='country',
timevar='year',
xvar='log_gdpPercap',
yvar='lifeExp',
colorvar='continent',
sizevar='pop_m')
plot(m)
Your Turn: Repeat the motion chart with the country having the highest gdpPercap
filtered out.
In github.com; we’ll walk through this together. Then, practice more; make changes to their repo, and to their neighbor’s.
Thematic maps tmap
:
library(tmap) # install.packages('tmap')
# load world spatial polygons
data(World)
# inspect values in World
World@data %>% tbl_df()
# gapminder countries not in World. skipping for now
g %>%
anti_join(World@data, by=c('country'='name')) %>%
arrange(desc(pop))
# World countries not in gapminder. skipping for now
World@data %>%
anti_join(g, by=c('name'='country')) %>%
arrange(desc(pop_est)) %>%
select(iso_a3, name, pop_est)
# join gapminder data to World
World@data = World@data %>%
left_join(g, by=c('name'='country'))
# make map
m = tm_shape(World) +
tm_polygons('lifeExp', palette='RdYlGn', id='name', title='Life expectancy (years)', auto.palette.mapping=F) +
tm_style_gray() + tm_format_World()
m
# show interactive map
tmap_leaflet(m)